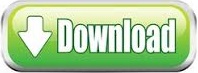
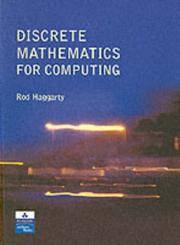
For this research, we created two CHI datasets, the CHI-I containing 171 color images of the cervical histology microscopy and CHI-II containing 1049 extracted fragments of microscopy, which are the most considerable publicly available SSE datasets. The design brings significant improvement to the identification of diagnostically significant regions. Further classification of the extracted epithelial fragments based on DenseNet made it possible to effectively identify the SSE RoI in cervical histology images (CHI). This paper proposes a novel approach for epithelium RoI identification based on automatic bounding boxes (bb) construction and SSE extraction and compares it with state-of-the-art histology RoI localization and detection techniques.

Hence, taking the entire image for classification adds noise caused by irrelevant background, making classification networks biased towards the background fragments. The typical RoI is the stratified squamous epithelium (SSE) that appears on relatively small image areas. The region of interest (RoI) identification has a significant potential for yielding information about relevant histological features and is imperative to improve the effectiveness of digital pathology in clinical practice. EpithNet-mc model, formed by intermediate concatenation of the convolutional layers of the three models, was observed to achieve 94% Jaccard index (intersection over union) which is 26.4% higher than the benchmark model.ĮpithNet yields better epithelial segmentation results than state-of-the-art benchmark methods. The proposed model was evaluated on 311 digitized histology epithelial images and the results indicate that the technique maximizes region-based information to improve pixel-wise probability estimates. EpithNet employs three regression networks of varying dimensions of image input blocks (patches) surrounding a given pixel, with all blocks at a fixed resolution, using varying network depth. This article presents EpithNet, a deep learning approach for the critical step of automated epithelium segmentation in digitized cervical histology images. Automated pathology techniques for detecting cervical cancer at the premalignant stage have advantages for women in areas with limited medical resources.
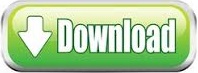